Frankly Speaking, 4/16/19 -- Undefined Behavior in Code Development
A weekly(-ish) newsletter on random thoughts in tech and research. I am an investor at Dell Technologies Capital and a recovering academic. I am interested in security, blockchain, and devops.
This past week, I did a podcast on my life as a VC and reflect on my time as a PhD student at MIT. I also talk about the surprisingly natural transition from academia to VC, and explain my worry that the human and people aspect of tech is being lost.
As always, if you were forwarded this newsletter, you can subscribe here.
WEEKLY TECH THOUGHT
This week, I’ll be writing about potential security issues that might occur in the code development cycle. This work was done by Xi Wang, who is an all-star computer science professor at University of Washington and a good friend. A lot of his work surrounds building secure and reliable systems, so if this article interests you, I encourage you to check out his other work.
There is a common belief that compilers like GCC are faithful translators of code to x86. However, this isn’t true if your code invokes undefined behavior, and there are serious security implications as result. For example, consider the piece of code below.
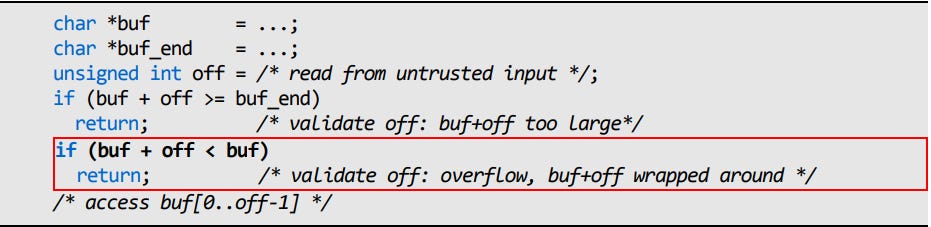
The C specification says that pointer overflow is undefined behavior. In GCC, (buf + off) cannot overflow, but that’s different from the hardware! From the compiler’s perspective,
if (buf + off < buf) == if (false)
So, it would delete that x86 code as an optimization. To attack this, an adversary would construct a large value for off and trigger a buffer overflow.
The fundamental problem is that undefined behavior allows such optimizations to happen. So, what exactly is undefined behavior? Undefined behavior is a spec that imposes no requirements. The original goal was to emit efficient. However, compilers assume a program never invokes undefined behavior. For example, if no bound checks are emitted, assume no buffer overflow.
Here are some examples of undefined behavior in C:
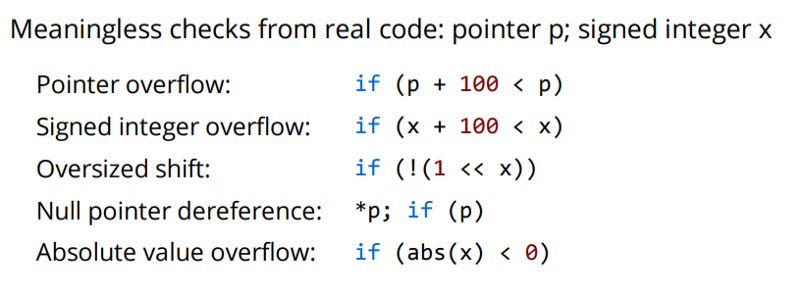
However, the problem is that undefined behavior confuses programmers. It produces unstable code, which is code that could be discarded by the compiler because of undefined behavior. As a result, security checks can be discarded, weaknesses are amplified, and system behavior becomes unpredictable.
Xi did a case study of unstable code in the real world, creates an algorithm for identifying unstable code, and wrote a static checker STACK, which found and fixed 160 previously unknown bugs.
Let’s take a look at another example of a broken check in Postgres. Say we want to implement 64-bit signed division x/y in SQL:

We place the check above, but some compiler optimize away the check. x86–64 idivq traps on overflow, leading to a DDoS attack.
This is Xi’s proposed fix, which is compiler-independent:

This is the developer’s fix:

However, that fix is still unstable code.
Here are some observations from his work:
Compilers silently remove unstable code
Different compiler behave in different ways, so changing /upgrading compilers could lead to a system crash
Need a systematic approach to finding and fixing unstable code
Their approach is to precisely flag unstable code: C/C++ source → LLVM IR → STACK → warnings
Here is the design overview:
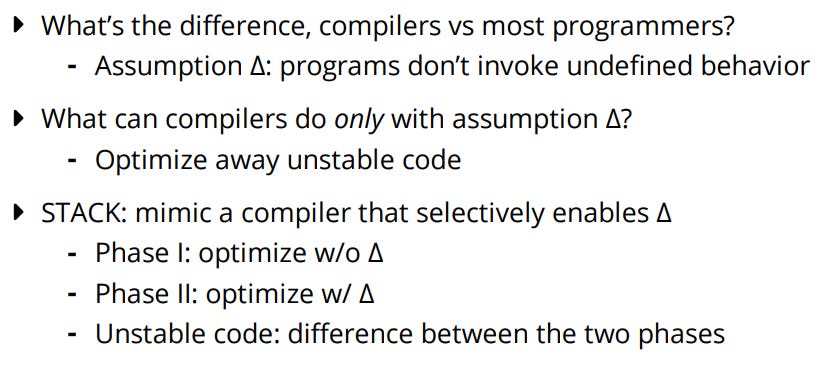
They were able to find 160 new bugs with low false positive rates. Similarly, STACK was able to scale to large code bases and generate warnings in a reasonable amount of time.
If you’re interested in the bugs they found or for technical details on their approach, I encourage you to check out their paper. You can also find his code here.
This is an interesting piece of work that shows sometimes security bugs occur unintentionally because compilers or language developers make incorrect assumptions about programmer’s behavior. It’s hard to avoid these bugs, but having development tools for detecting is important!
For those who have further interest, consider the following question:
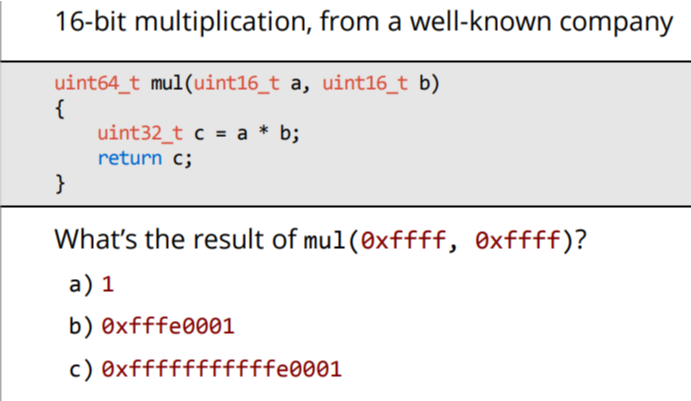
WEEKLY TWEET
Let's take a break with our weekly tweet before I get into my weekly Frank Thought
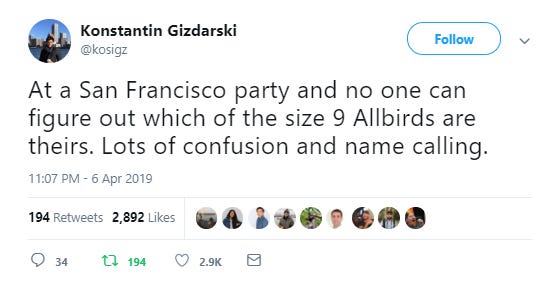
And this is why I don't wear Allbirds.
WEEKLY FRANK THOUGHT
I expressed my frustration over tech's obsession and lack of rigor in their handling of data. Coincidentally, this week's Defense in Depth discusses machine learning's failures. This week, I want to do a more technical evaluation of biases in data. Most of my content is based on a paper by Harini Suresh, a MIT PhD student in machine learning. Because of space and time, I will only discuss a couple of the biases and then continue next week.
There are five main sources of bias in AI/ML: historical bias, representation bias, measurement bias, aggregation bias, and evaluation bias.
Historical bias occurs if the data collection and measurement process is done perfectly. This bias occurs because of a simple problem: The past isn't always representative of the future. More philosophically, Winston Churchill said that history is written by the victors. A good example is crime data. A perfectly sampled and measured crime dataset might show more crime in poorer neighborhoods, but this might also reflect historical factors leading to these "facts." Another example is that about 5 percent of CEOs in the Fortune 500 companies are women. Should an image search reflect this "fact"? One of the main consequences of historical bias is that it tends to attribute negativity or reflect harm to a specific identity group. Sometimes, it's possible to provide context, but many times, the context is lost or complex.
Representation bias occurs because one group is under-represented in the input dataset. There are many causes for this. A couple of key reasons are:
1. Only a specific population is sampled.
2. The population that model is evaluated on is distinct from the population that the model was trained on.
For example, if you train a model based on cars in the US, that model might not perform as well in Europe because European cars tend to be smaller and shorter.
There might not be good ways to eliminate these biases, such as historical biases, but it is important to be aware of them. I am really trying to challenge the notion that "data are facts." Our interpretation of data and facts should be aware of potential biases. Accepting data on face value is dangerous!
FUN NEWS & LINKS
#securityvclogic
“We missed the last 4 big companies in the vulnerability management space, so we have to invest in the next company we see to show our LPs we are trying to be active in security.”
#research
Black hole picture by MIT students!
Hybrid long haul trucks.
#tech, #security, #vclife
Family tracking app leaking location data.
Amazon's growth is slowing!
Random Forests and Decision Trees for beginners.